Back in September last year I blogged about the implications for cultural heritage and digital humanities crowdsourcing projects that used simple tasks as the first step in public engagement of advances in machine learning that mean that fun, easy tasks like image tagging and text transcription could be done by computers. (Broadly speaking, 'machine learning' is a label for technologies that allow computers to learn from the data available to them. It means they don't have to specifically programmed to know how to do a task like categorising images – they can learn from the material they're given.)
One reason I like crowdsourcing in cultural heritage so much is that time spent on simple tasks can provide opportunities for curiosity, help people find new research interests, and help them develop historical or scientific skills as they follow those interests. People can notice details that computers would overlook, and those moments of curiosity can drive all kinds of new inquiries. I concluded that, rather than taking the best tasks from human crowdsourcers, 'human computation' systems that combine the capabilities of people and machines can free up our time for the harder tasks and more interesting questions.
I've been thinking about 'ecosystems' of crowdsourcing tasks since I worked on museum metadata games back in 2010. An ecosystem of tasks – for example, classifying images into broad types and topics in one workflow so that people can find text to transcribe on subjects they're interested in, and marking up that text with relevant subjects in a final workflow – means that each task can be smaller (and thereby faster and more enjoyable). Other workflows might validate the classifications or transcribed text, allowing participants with different interests, motivations and time constraints to make meaningful contributions to a project.
The New York Public Library's Building Inspector is an excellent example of this – they offer five tasks (checking or fixing automatically-detected building 'footprints', entering street numbers, classifying colours or finding place names), each as tiny as possible, which together result in a complete set of checked and corrected building footprints and addresses. (They've also pre-processed the maps to find the building footprints so that most of the work has already been done before they asked people to help.)
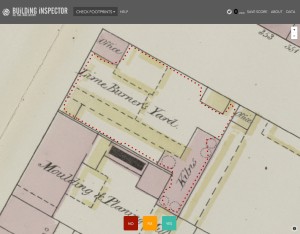
After teaching 'crowdsourcing cultural heritage' at HILT over the summer, where the concept of 'ecosystems' of crowdsourced tasks was put into practice as we thought about combining classification-focused systems like Zooniverse's Panoptes with full-text transcription systems, I thought it could be useful to give some specific examples of ecosystems for human computation in cultural heritage. If there are daunting data cleaning, preparation or validation tasks necessary before or after a core crowdsourcing task, computational ecosystems might be able to help. So how can computational ecosystems help pre- and post-process cultural heritage data for a better crowdsourcing experience?
While older ecosystems like Project Gutenberg and Distributed Proofreaders have been around for a while, we're only just seeing the huge potential for combining people + machines into crowdsourcing ecosystems. The success of the Smithsonian Transcription Center points to the value of 'niche' mini-projects, but breaking vast repositories into smaller sets of items about particular topics, times or places also takes resources. Machines can learn to classify source material by topic, by type, by difficulty or any other system that crowdsourcers can teach it. You can improve machine learning by giving systems 'ground truth' datasets with (for example) a crowdsourced transcription of the text in images, and as Ted Underwood pointed out on my last post, comparing the performance of machine learning and crowdsourced transcriptions can provide useful benchmarks for the accuracy of each method. Small, easy correction tasks can help improve machine learning processes while producing cleaner data.
Computational ecosystems might be able to provide better data validation methods. Currently, tagging tasks often rely on raw consensus counts when deciding whether a tag is valid for a particular image. This is a pretty crude measure – while three non-specialists might apply terms like 'steering' to a picture of a ship, a sailor might enter 'helm', 'tiller' or 'wheelhouse', but their terms would be discarded if no-one else enters them. Mining disciplinary-specific literature for relevant specialist terms, or finding other signals for subject-specific expertise would make more of that sailor's knowledge.
Computational ecosystems can help at the personal, as well as the project level. One really exciting development is computational assistance during crowdsourcing tasks. In Transcribing Bentham … with the help of a machine?, Tim Causer discusses TSX, a new crowdsourced transcription platform from the Transcribe Bentham and tranScriptorium projects. You can correct computationally-generated handwritten text transcription (HTR), which is a big advance in itself. Most importantly, you can also request help if you get stuck transcribing a specific word. Previously, you'd have to find a friendly human to help with this task. And from here, it shouldn't be too difficult to combine HTR with computational systems to give people individualised feedback on their transcriptions. The potential for helping people learn palaeography is huge!
Better validation techniques would also improve the participants' experience. Providing personalised feedback on the first tasks a participant completes would help reassure them while nudging them to improve weaker skills.
Most science and heritage projects working on human computation are very mindful of the impact of their choices on the participants' experience. However, there's a risk that anyone who treats human computation like a computer science problem (for example, computationally assigning tasks to the people with the best skills for them) will lose sight of the 'human' part of the project. Individual agency is important, and learning or mastering skills is an important motivation. Non-profit crowdsourcing should never feel like homework. We're still learning about the best ways to design crowdsourcing tasks, and that job is only going to get more interesting.
Building on some comments shared on Twitter:
Bringing human machines and machine machines into the #welearntogether mix, I'm more than enthused by the suggestion that connecting the tiniest of tasks in related spaces can create paths to improve data and experiences. Break it down, build up participants (in spirit and quality, and perhaps even quantity), (re)connect it, and watch it grow!
What I'd like to see next: many things – but most of these move past building the case and example of how and why to crowdsource. Now is the time to work harder, faster, and more openly to connect the knowledge developed in "first encounters" with projects to new, evolving (or spinoff!) tasks on same material. Then we can collaboratively leverage the human systems, organizational infrastructures (and cultures), and technologies to improve access to knowledge and the experiences associated with that access – all while creating spaces that combine opportunities for amateur & expert knowledge (that baggage we bring with us whether we know or are being introduced to a project) to be stored together and regularly unpacked. Also now is the time to match data and learning generated in projects to other tasks that transcend project/system borders; i.e. "what can the ways folks transcribe TC diaries do to help improve NYPL map marking, & vice versa."
My experience suggests (shows) participants want to help each other, themselves, & the system do it better.
Also, an additional ecological layer or systems observation for the Smithsonian Transcription Center may be the connectedness of subject/period/place/people/concepts – each segmented project stems from physical arrangement and categorization relationships (suggesting it might pull niche audiences, too) – YET, we always see more activity on site and movement between projects when we have a wider variety of material types (despite having "only" transcription and data extraction as tasks). Hoping to explore and share more analysis of this soon.
Thanks for this excellent post, Mia!